How to read a forest plot
- Mar 3, 2021
- 3 min read
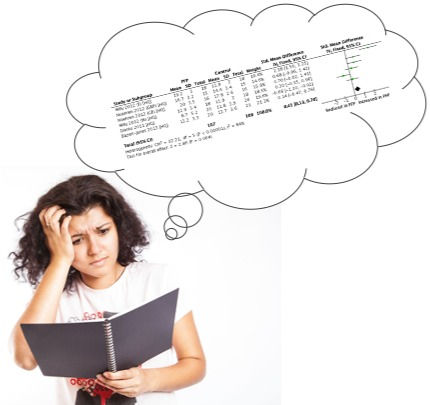
Systematic reviews & meta-analyses are great. When well conducted, they literally do the work for you. They take data from several studies, mix it all together and
finish by giving you a level of evidence which reflects a statistical conclusion from a group of comparable studies.
Yet, whenever we teach our PFP course and ask how many people are comfortable interpreting a forest plot, most participants shake their head. This post is designed to get clinicians more comfortable with reading and interpreting forest plots, which is where the gold lives in a systematic review.
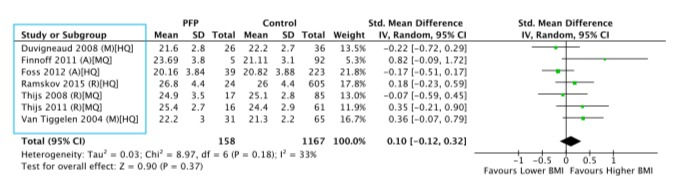
We should now know how many studies are included and their associated quality. Next, we need to understand what type of data we are dealing with:
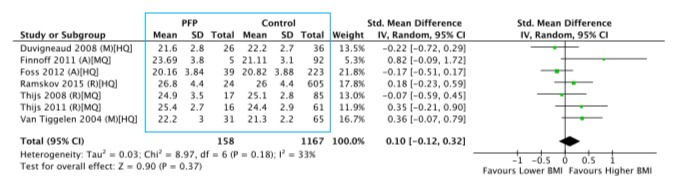
Here, we can see the mean, standard deviation (SD) and total number of participants for each group, in this case those who developed PFP and those who remained asymptomatic (the control group). These data refer to participant BMI and are an example of continuous data (i.e. data that can take any value within a range, but are essentially infinite).
Now, we can see the result of mixing these data together.

This means lots of numbers, but bear with us. Each study has its own standardised mean difference (SMD), which is calculated by taking the difference in the group means and dividing by the standard deviation of the groups. There are also associated 95% confidence intervals.
The most important number to look at here is the pooled SMD, which is in bold underneath the individual study SMDs. Look at the confidence intervals, if they cross zero (i.e. one is negative, one is positive) as they do here, the outcome is non-significant. This can be especially useful when looking at forest plots comparing two treatment approaches, but can mean different things dependent on the research question.
There are two primary types of meta-analysis model used - fixed or random. This plot was produced using a random effects model, which in short means that we assume no directionality of the outcome (in this case, we don't anticipate those who develop PFP to have a higher or lower BMI). This model should be the preference for most musculoskeletal meta-analyses, as we generally deal with small numbers of studies (<20) and should assume both the absence of directionality the potential for heterogeneity. As a result, there is one more number you should look at:
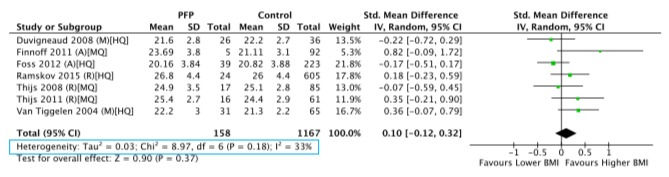
I2 is a measure of statistical heterogeneity (difference). Don't get bogged down by this, but we do need to factor it in to out level of evidence. If I2 is above 50%, the outcome is heterogeneous and the level of evidence cannot be strong. Another tip is that if I2 is below 50% and data are homogeneous, P will be greater than 0.05, which translates as 'these data are not significantly different'.
To recap, you should now be able to determine how many studies are included in an outcome and their quality, what type of data are involved, if the pooled SMD is significant and what model was used to produce it and if the outcome is homogenous. If there are at least three high quality studies and data are homogeneous, strong evidence is likely to be the conclusion.
Can we make it even easier?
We can. Just look at the pretty picture:
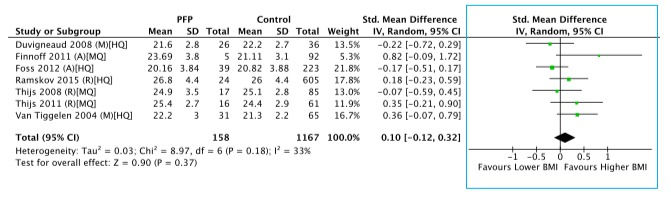
If you want to avoid the numbers or skim through an outcome, just look at the black diamond. This is a visual representation of the pooled SMD and its confidence intervals and if it touches the centre line, the outcome is non-significant (i.e. the confidence intervals cross zero). The further away from the centre line the black diamond is, the larger it is (but beware, it may be stretched out, which represents a wider confidence interval).
The green squares represent each studies individual SMD and the extending lines the confidence intervals. The larger the green square, the greater the weight of the study in the pooled SMD. The further away from the centre line it is, along with its weighting, tells you how much a single study may be skewing the pooled outcome.
Take home messages
Systematic reviews can be daunting, but they needn't be. We hope that you now feel in a position to comb the results of a review in greater depth and have a greater understanding of the statistics that contribute to an eventual level of evidence.
However, if you want to know if an outcome is significant or not, keep it simple and look at the black diamond on the plot. You can always delve deeper at a later date.
Happy reading!
Yorumlar